AI on Camera
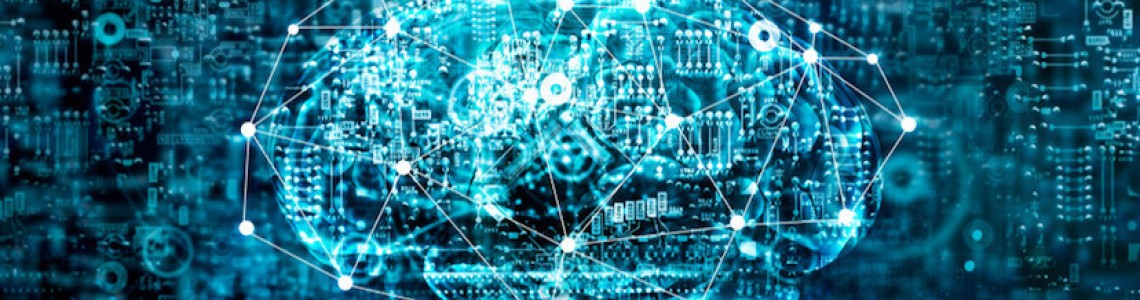
When you think of artificial intelligence (AI), what springs to mind? For some, it’ll be the vast server rooms that power the likes of IBM Watson or AlphaGo. But that’s no longer the case when deploying AI at the edge. With AI analytics being done by devices situated throughout our physical world, not all AI actions require streaming data back to a server. Which explains why the market is predicted to grow at a compound annual growth rate of 19.27% from 2019 to 2026.
AI at the edge promises a host of benefits for network surveillance systems — let’s run through the top five most impactful for installers and end users.
1. More accuracy and context
Running AI on a device can improve the accuracy of triggers and reduce false alarms. People counting, occupancy measurement, queue management, and more, can all be carried out with a high degree of accuracy thanks to the edge AI using deep learning. Operators will also get greater insights from edge devices, such as contextual information like if someone is wearing glasses or carrying and bag, and vehicle colour.
2. Cost of ownership decreases
Globally it’s estimated that security cameras generate around 2,500 petabytes of data per day. Sending all of this data back for storage and analysis is costly, so doing AI at the edge will immediately save the cost of data transfer (as only critical data and metadata needs to be sent back), plus maintaining expensive servers. This also leads to energy savings.
3. Latency improvements
As Thomas J. Bittman, Distinguished VP Analyst at Gartner explains, “As people need to interact with their digitally-assisted realities in real-time, waiting on a data center miles (or many miles) away isn’t going to work. Latency matters. I’m here, right now, and I’m gone in seconds.”
With analytics done at the edge, triggers and alerts are more immediate. That improves the speed of response when dealing with an event, such as trespassing, and the overall experience of accessing and moving around a site or entering a car park.
4. Greater scalability
Cameras using edge AI will make a video installation more flexible and scalable as more cameras and devices can be added as and when needs evolve (without committing to a large server with significant bandwidth from the start). This is particularly helpful for organisations wishing to deploy a project in stages.
5. Reliability
With analytics being done across different devices, if one fails, another can take over. There is no single point of failure. The AI can continue to operate even if a network or cloud service fails. Triggers can still be actioned, access can still be granted, and so forth, with local recordings and insights sent to the back-end when connections are restored.
Gaining an edge
This is the perfect time for end-users and installers to explore AI at the edge for their systems because of the huge efficiency, accuracy, and sustainability gains that it promises. Like any innovation, start with your goals and use case, and then test the waters — which edge AI is perfect for since it can be deployed in stages.